Using Benchmarks to Explore Population Health Data
This feature was originally published on the IP3 Blog and is being reproduced for Community Commons with permission of the Institute for People, Place, and Possibility.
Using Benchmarking When Exploring Population Health
It is well established that assessing a community before developing and prioritizing strategies to advance community well-being is important. This process often involves exploring qualitative data (e.g., community listening sessions) and quantitative data (e.g., population health indicators) —often referred to as Community Health Assessment or Community Needs Assessment. These assessments are important because they help changemakers account for local community conditions when planning to improve the community rather than generalizing or making assumptions about what is needed. When exploring quantitative data about a given community, benchmarks serve as helpful comparative references—added context through benchmarking tells us more about how a community is really doing.
Benchmarks Help Us Interpret Population Health Data
Benchmarks serve as points of reference and give context to help us interpret what we see in the data. Without benchmarks, it can be difficult to draw conclusions from data. What can we make of the fact that the rate of infant deaths per 1,000 live births is 5.87 in San Bernardino County, for example, without a benchmark for comparison? Without benchmarks, it’s difficult to know where to focus efforts and how to prioritize investments to improve community conditions. Of course, we can always work toward improvement, but strategic plans and collaborative efforts are often met with scarce resources—benchmarks provide context for what we’re seeing so we can make better decisions about where to strategically act and invest together.
When considering how to use benchmarks for comparative reference in a given community, there are a number of factors to consider:
-
Sourcing relevant data: In order to consider a particular benchmark, one must access comparable data for the benchmark area. For example, in order to compare San Bernardino County, California to the state as a whole, one must access data for the entire state. If one wishes to make a national comparison, the same data must be available across the U.S. In short, before selecting benchmark(s), it’s important to ensure data availability.
- Selecting an appropriate benchmark: In order to identify areas of concern or priority for action in a community, it’s helpful to consider geographic context. For example, one would expect the rate of infant deaths in most of California to be better than the national average because California as a state typically performs better than much of the rest of the U.S. on population health indicators. Therefore, choosing a national benchmark would not be as meaningful as a state or peer community.
- Using more than one benchmark: The more context one has for interpreting data, the more meaning one can glean, so when possible, use multiple benchmarks to triangulate findings. When dealing with population health data, state and national averages are often used as benchmarks, but others might work well for your community and project. It often makes sense to compare data from two neighboring communities or even two communities that aren’t contiguous but have similar populations, demographics, and community conditions. Similarly, regional benchmarks can offer additional nuance. One might expect data from two similar communities to be similar, so identifying specific areas where one community fairs better or worse than the other could shed light on “low-hanging fruit” strategies for community improvement. Other commonly used benchmarks include well-established goals, like or the UN Sustainable Development Goals
Using Benchmarks To Explore Data in San Bernardino County
In San Bernardino County, California, a group of stakeholders is examining data around maternal and infant health to determine how the county fairs today and what might be done to improve maternal and infant health in the region. One key indicator is Early Prenatal Care (the percent of births for which prenatal care began in the first trimester), a dataset made available through CDC WONDER. Early prenatal care is important because it reduces risk of pregnancy and birth complications and is associated with better maternal and infant health outcomes. In San Bernardino County, the percent of births with early prenatal care is 84.2%, which is higher than the national average (benchmark) of 77.6%, lower than the California state average (benchmark) of 85.5%, and just slightly lower than the neighboring (peer) community of Riverside County.
As you can see, examining benchmarks alongside data for San Bernardino County provides a more complete picture of how the community fairs when it comes to early prenatal care. We can infer that San Bernardino County provides better access to prenatal care than most communities in the U.S., but that there is still room for improvement compared to the rest of the state, and thus increasing early prenatal care as a means to improve maternal and infant health is likely worth prioritizing in San Bernardino County.
Key Considerations When Using Data Benchmarks
Benchmarks can serve as goals but should not be confused with goals—just because your community is faring better than the state or nation when it comes to the rate of infant deaths, for example, doesn’t mean there isn’t still a need for improvement. Benchmarks provide useful context to aid in interpreting population-level data, whereas goals are established objectives that, when reached, indicate a level of success in improving population health. Ideally, population health goals are established and worked towards collectively—among stakeholders, community partners and organizations, and residents.
Benchmarks do not always shed light on disparities. Where possible, it is best to examine data (and associated benchmarks) broken out by race, ethnicity, and other population groups. See the example below:
Overall, San Bernardino County is performing the same as the national benchmark when it comes to learning rate (the average grade-to-grade level improvement in test scores within each cohort for students in grades 3-8). However, when we explore data by race and ethnicity, it’s clear that among children in poverty, Black and African Americans, Hispanic and Latinx people, American Indian and Alaskan Native people there is much room for improvement. In this case, looking at the data broken out by population groups exposes important opportunities to support specific priority populations to decrease disparities and increase the learning rate overall.
A limitation to using state and national benchmarks is that when we lack data for some geographies we aren't using a truly representative benchmark. This is relevant for datasets for which national and/or state data may be incomplete or spotty.
Related Topics
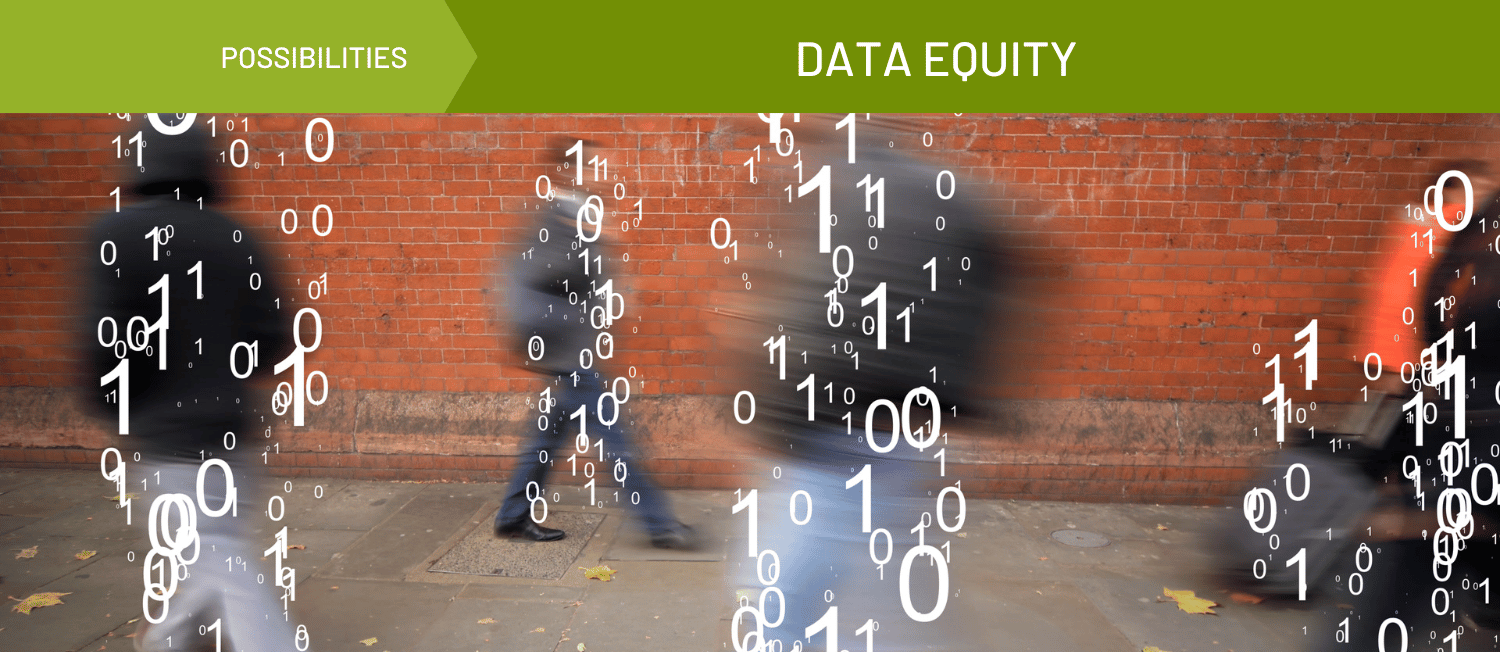
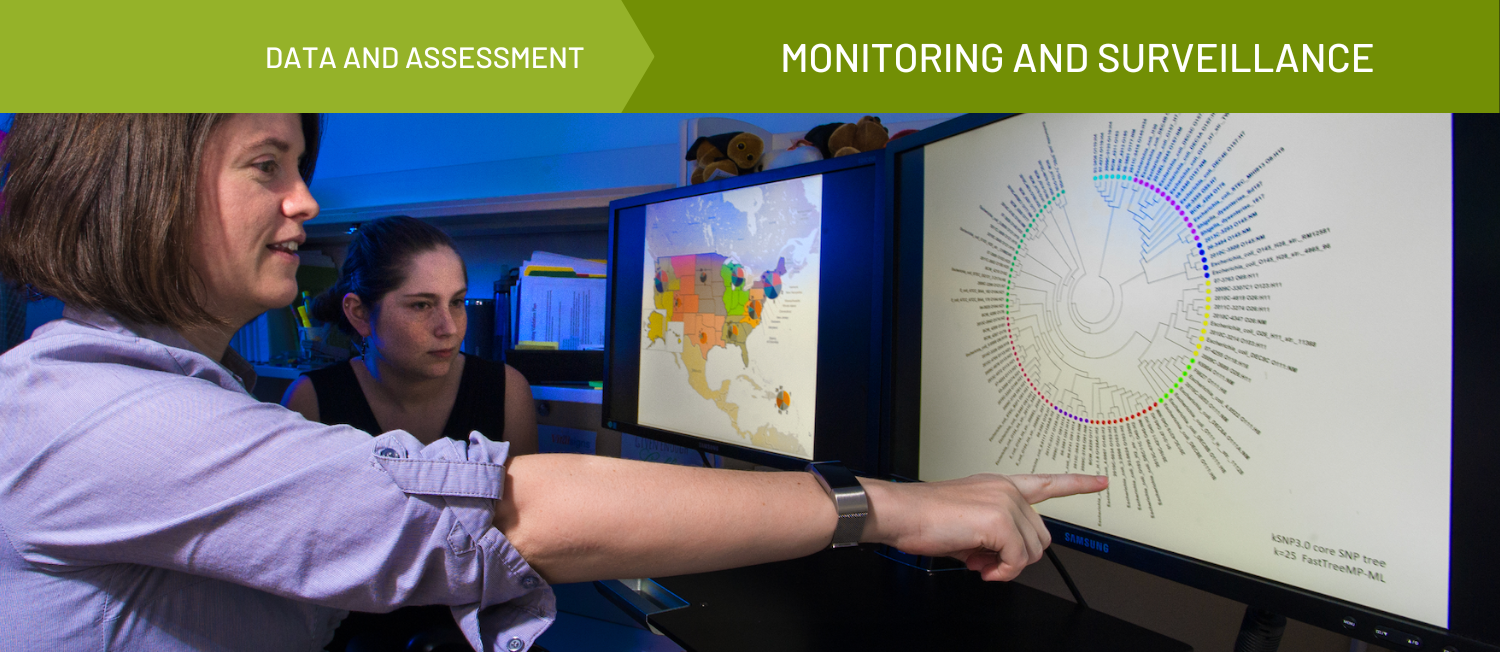
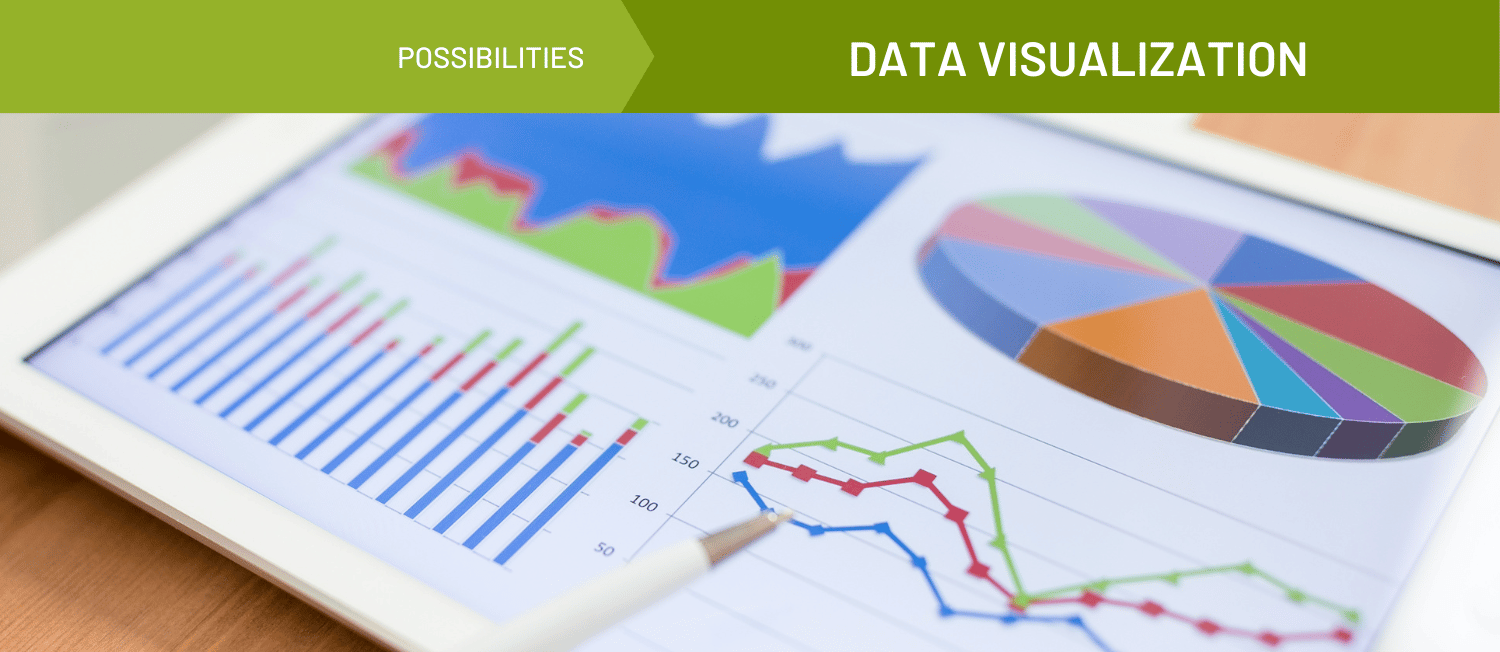
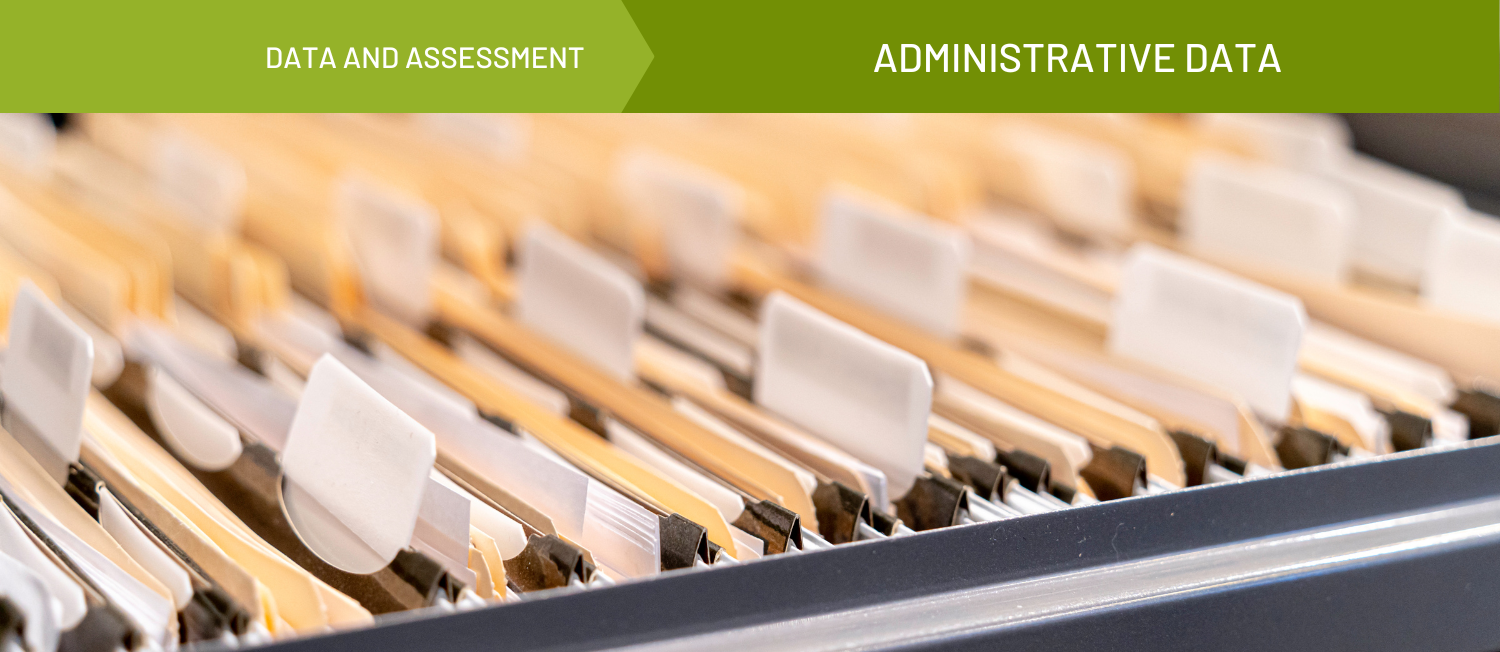
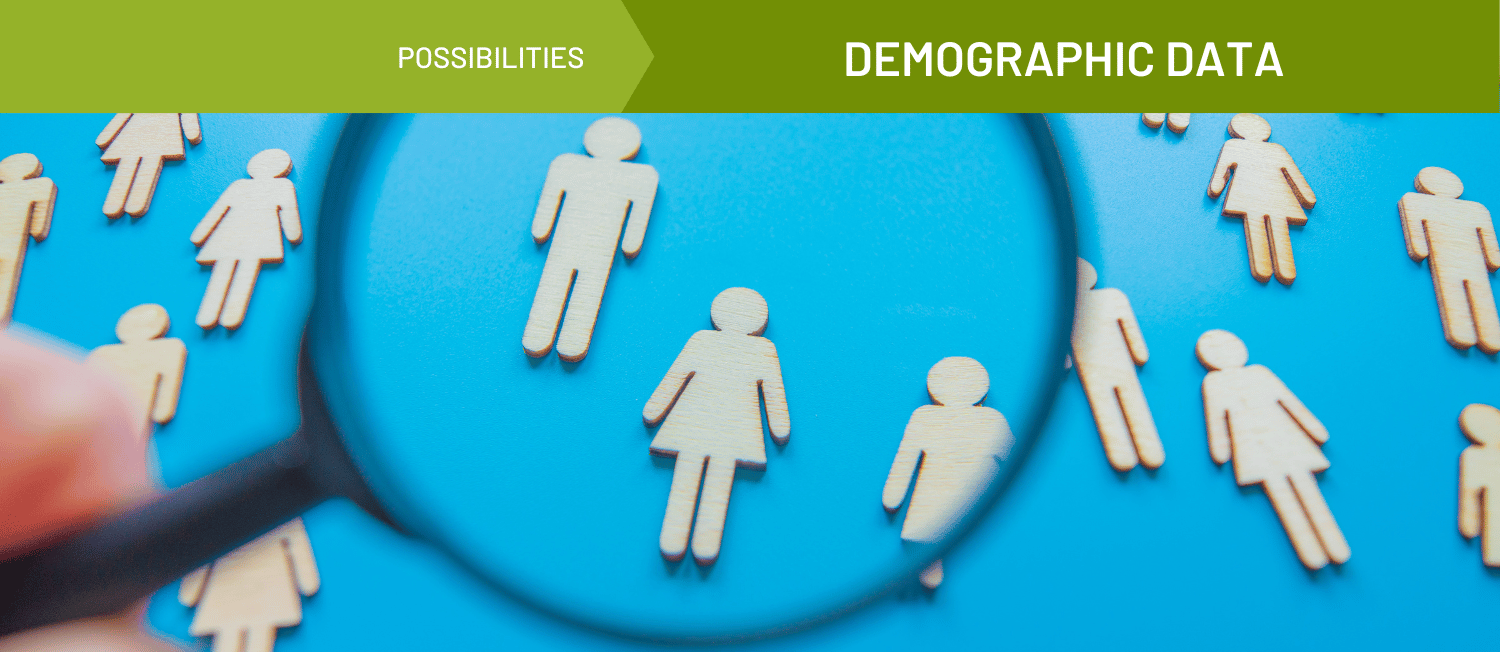
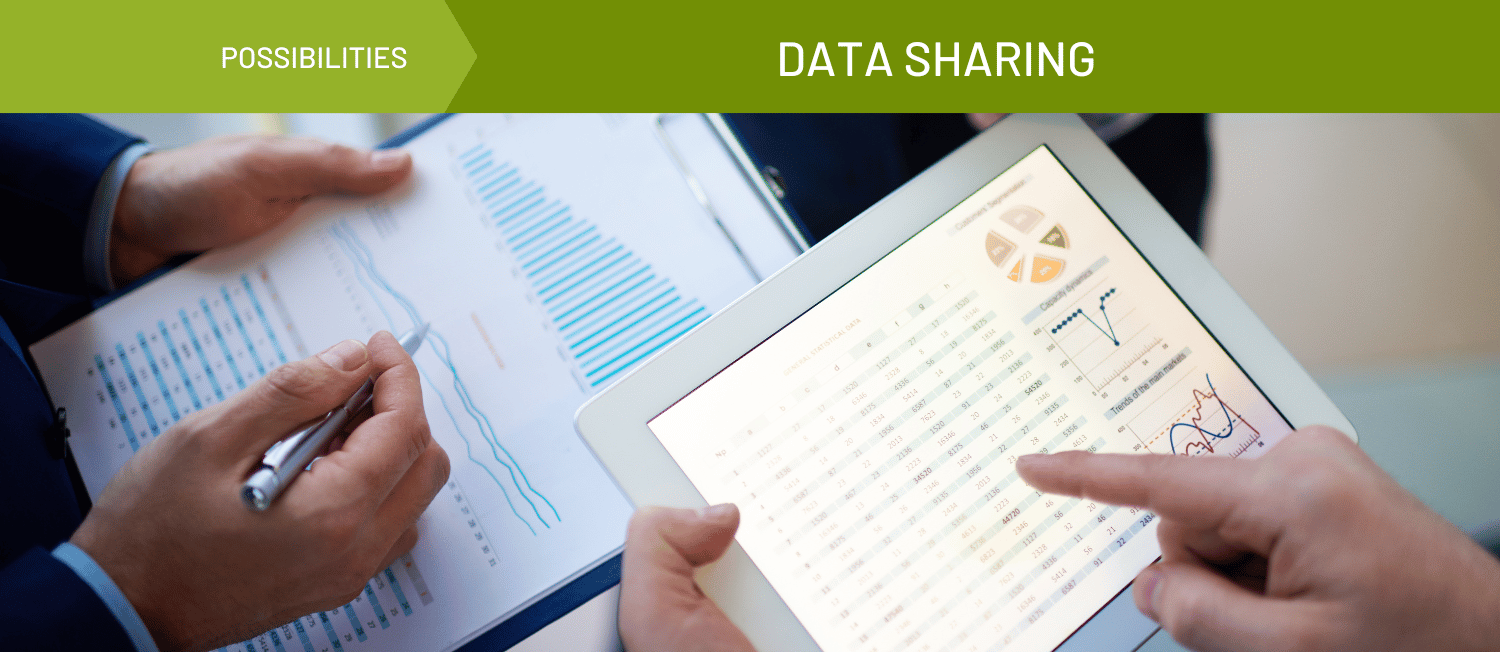